Regardless of your stated approach to AI in your class, there are still trends and attitudes in the larger world or simply in other educational settings that stand to have an impact on the environment you want to cultivate. Norms from other classes regarding AI and even counterproductive habits of use can come with students into your classroom and potentially come up against your teaching goals–especially when it comes to emerging technology like generative AI, where the rules vary so greatly. In the first post in this series, we presented a broad overview of three general dispositions toward AI in higher education (teaching against, teaching around, and teaching with), accompanied by a few illustrative examples.
In this next post, we present a few more frameworks to help you better contextualize your teaching and even potentially consider new ideas to incorporate into your work. Generative AI in teaching can be a fuzzy mess of issues, but with the help of the right lens, some of the lines you want to draw between helpful and harmful uses can acquire greater definition.
Framework Two: “Bloom’s Taxonomy, Revisited”
Most educators are familiar with Bloom’s Taxonomy, the hierarchy of learning and cognitive tasks that conceives of student learning as moving up in difficulty and complexity from foundational tasks like memorization of facts to higher-order work like application of concepts and synthesis of ideas. The Vanderbilt Center for Teaching’s excellent graphic demonstrating the pyramid building from “remember” all the way up to “create” will likely be familiar to most teachers.
Image credit: Vanderbilt University Center for Teaching
Even with some revision and iteration over the years, Bloom’s taxonomy has long been a useful approach to conceiving of appropriate tasks for the students’ level. For an example of how that’s been well employed, check out the Chicago Center for Teaching and Learning’s Resource on Asking Effective Questions. The example above from Vandebilt reflects a 2001 update of the original 1956 version, which rearranges the order of higher-level tasks slightly and changes the categories from nouns to actions in order to emphasize what students do.
An additional update proposed by Oregon State University suggests how Bloom’s Taxonomy could be reconsidered in light of generative AI’s disruption of learning and assessment in education. This revisitation offers recommendations for review or amendment of each level, a description of the capabilities AI has relevant to it, and reflections on the human skills that remain distinct from what AI tools can currently do.
Image credit: Oregon State University
In this example, you’ll find learning tasks easily circumvented by AI like “describing concepts” paired with uniquely human tasks like emotional or ethical commentary on the concept described. Head over to Oregon State’s website to review in depth.
Framework Three: The Four Ds
Originally presented at Stanford as the Three Ds, the Four Ds have been popularized by EDUCAUSE as a way of thinking of distinct types of tasks that come up in higher education teaching and learning. This framework is also a good reflection of how AI is starting to be conceived of in the world of knowledge work in general, so it may be a useful tool for thinking about how students may be called upon to use these tools in the working world–and even in classes other than your own. Even when you prefer to keep AI out of your class, this context can help you plan to support your course design.
In conversations about AI being employed by a discerning user to generate, evaluate, and apply outputs, you may sometimes hear it described as a “force multiplier,” by experts such as Ethan Mollick at the Wharton School of Business. You’ll see that this framework reflects a similar outlook.
- Dreaming: Helping You Think
- Drudgery: Lightening Your Load
- Design: Building Your Content
- Development: Advancing Your Work (added by EDUCAUSE)
Image credit: EDUCAUSE
Dreaming: Helping You Think
This part of the framework includes using AI for things like idea generation and overcoming writer’s block. For example, a student might type into a generative AI tool “Give me ten ideas for a paper about Thomas Kuhn’s concept of paradigms’ relevance to different fields.” Or, an instructor might write “Give me five analogies I can use to explain how inflation works.” From there, the user can assess the results and consider what idea merits pursuing in depth on their own.
Drudgery: Lightening Your Load
Think of this category as being like a very advanced spell check or autocomplete function, in which users generate highly formulaic content by prompting for the predictable parts of a document, providing relevant specific details to be filled in, and then customizing as desired.
Design: Building Your Content
This category encompasses more creative and collaborative uses of generative AI tools. In education, this might include designing simulations, personalized learning experiences and customized lesson ideas. Some users might even use an AI tool to help find and build out areas where their course could be more accessible.
Development: Advancing Your Work
This category, added to the original Stanford framework by EDUCAUSE, features use cases like strategic planning, where the user might work with a generative AI to map out steps to a larger goal, considering strengths, weaknesses, opportunities, and threats to success. They can provide a goal and some context, talk through details. and prompt for drafts formatted in an approachable style by the tool for further revision.
A Final Frame: AI as a “Camera”
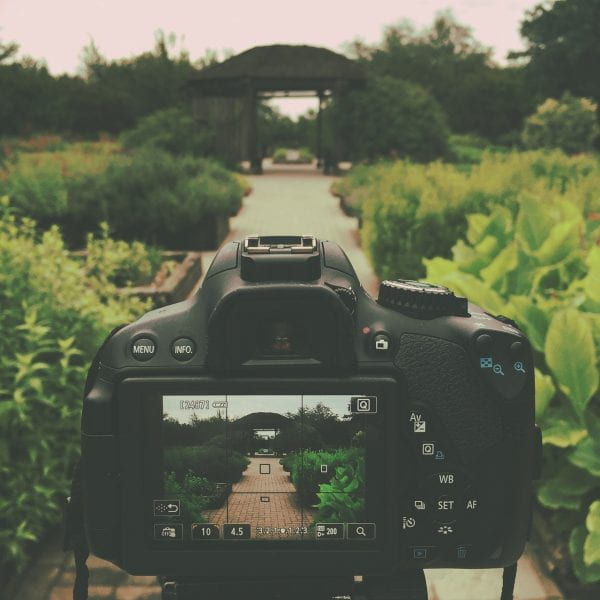
Image by Dionne Hartnett from Pixabay
The original workshop that inspired this post was initially titled “Can AI Be a Camera for Learners?,” and that much simpler and broader metaphor closes out our review of frameworks for your consideration. The metaphor comes originally from Derek Bruff, the author of books like Intentional Tech and host of the podcast Intentional Teaching. Bruff’s reflection on the camera as a learning tool provides a useful way to “frame” (so to speak) a variety of applications for generative AI tools and explore how they might help students think through problems actively:
“When I was learning photography, there was a lot of conceptual learning about light and depth of field and composition but also learning how to use my digital camera, what all the knobs and buttons did. As I experimented with taking pictures, my use of the camera helped sharpen my understanding of the relevant concepts of photography. And my better understanding of those concepts in turn informed the ways I used the knobs and buttons on the camera to take better photos.
…learning to write with AI tools might be like learning photography with a DSLR camera, where the conceptual learning (light, motion, composition) is enhanced by the technical learning (figuring out what all those knobs and buttons on the camera do).” (emphasis added)
A little more than a year into the wide availability of these tools, these various metaphors and categories will hopefully assist you in thinking about this massive change in ways that draw on your expertise as teachers to create learning experiences for this new situation.
If you found these useful, here are a few additional resources you may want to explore:
- Cynthia Alby’s AI Prompts for Teaching
- Ethan Mollick’s Assigning AI: Seven Ways of Using AI in Class
- Daniel Stanford’s Eight Categories for AI in Teaching for Busy Instructors
- Academic Technology Solutions’ own post on AI prompting to support learning
In Closing
For more ideas on this topic, please see our previous blog posts about generative AI. For individual assistance, you can visit our office hours, book a consultation with an instructional designer, or email academictech@uchicago.edu. For a list of our and upcoming ATS workshops, please visit our workshop schedule for events that fit your schedule. For support with training students in research skills, see our colleagues at the UChicago Library.